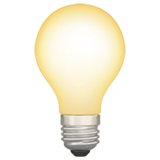
Article Snapshot
In 4,000 BC Babylonia, if you wanted to know how much food you needed to feed the population, you used statistics and analytics to draw conclusions from data captured on clay tablets in what is believed to be the very first census. Not exactly “big data” by our standards, but definitely big data in its day.
In about 2,500 BC, the ancient Egyptians also used data capture (another census) and analytical techniques to calculate the scale of workforce needed to build each pyramid.
It would be another 6,000 years before Microsoft finally released Excel in 1985, making the job of forecasting a famine-free future or planning the perfect pyramid project a little easier for the rest of us. (Ironically, the first version of Excel was designed for the Apple Macintosh, with a Windows version only coming out in 1987.)
But is this data science or data analytics? And which skill set do you need to meet your business’s current requirements?
Data analytics versus data science
Data science and data analytics are often spoken about interchangeably. However, while definitely related, they’re not quite the same thing; and the differences are often misunderstood.
Data science is more of an umbrella term to describe any practice used to manipulate or analyse data: making connections, identifying correlations, finding patterns and exploring new perspectives in search of previously unknown insights.
Data analytics, meanwhile, is a branch or subset of data science that seeks to test specific hypotheses, usually by measuring or benchmarking established criteria to predict trends or inform future decisions.
In short, data analysts use data to solve clearly defined problems or answer specific questions, while data scientists look for answers to questions you don’t even know to ask.
You might ask a data analyst to report on, “What’s our most profitable client segment?” which would require connecting and analysing two or more specific and clearly defined data sets. Meanwhile, you might ask a data scientist to explore, “How can we boost profitability of the business?” This latter question doesn’t specify which data may hold the answers, requiring the data scientist to sift through mountains of unstructured and raw data to create new data sets in the hope of finding previously unknown connections or trends.
If you’re still wondering why is data science important, this case study of a data science project might persuade you.
The role of a data scientist
While a data analyst may be confined to a particular department or capability within an organisation, such as the finance team, a data scientist needs to work with a much larger data set – in fact, any and all data available to the company.
Therefore, a good data scientist needs the access, as well as the business acumen, to understand all the interconnected parts of the business.
Almost any business over a certain size will already have some form of data analysis capability. All of those monthly reports and graphs don’t generate themselves. But that doesn’t mean you can just ask your data analyst to start being a data scientist. A data scientist may use a number of different tactics and tools to cleanse, categorise, connect, compare and crunch the mountains of raw or unstructured big data available within a typical business, many of which go far beyond the skills and capabilities of a typical data analyst.
For example, a typical data analyst probably won’t know how to code or how to build a statistical model. Most likely, they won’t have advanced programming or computer skills. After all, these skills aren’t necessary in most data analyst roles.
Another big difference is that data scientists also need to communicate their findings and insights in a way the rest of the business can understand, not simply provide a bunch of abstract statistics and spreadsheets. So a data scientist also needs to be a bit of a storyteller.The role of data scientist is far more specialised than you might think and may require you to source the expertise externally.
Preparing your data before you hire
Before embarking on the hunt for expert talent, businesses should ensure there is sufficient data integrity and completeness. There’s no point in bringing someone on who’s extremely expensive and qualified only to present them with an incomplete and inaccurate data set that can’t be relied upon. You don’t want to be paying someone $120,000 a year to clean up your databases and audit your systems – projects that can take weeks or months in themselves – before they can start on the scope of work you intended.
Instead, prepare ahead of the project. There are plenty of specialised data hygiene services and consultants out there who can make sure you’re ready to begin planning your first data science project.
Attracting the best data science talent
The demand for data science expertise is particularly hot right now, and that’s pushed up the day rate or the salary data scientists can command. Like engineers, the best data scientists are looking to solve the most challenging problems with, ideally, the most cutting-edge technology.
As a result, data scientists often prefer to seek out contract or project-based work because they want to jump around to whichever companies have the chunkiest problems to solve.
Spend a little time considering what challenges would make this initial project attractive, particularly to someone who may have solved so many similar problems before. Once a data scientist is engaged, they might suggest further opportunities or questions to explore as they learn more about your business.
Assessing the best data science talent
At times, high rates/salaries and time to onboard means hiring the wrong talent – perhaps a data analyst with delusions of grandeur – can be a potentially expensive mistake. So, you need to find ways to appropriately assess the skills and expertise of any data science talent you’re hoping to bring in.
One way is to have a case study for them to tackle in the interview process. Throw out a problem, give them a data set, and ask them to talk you through how they would approach solving that.
How would they crunch the data? How would they question the integrity of the data? And how would they pull their findings together at the end to present them to the leadership team? You’ll soon find out if they don’t know what they’re talking about.
Retaining the best data science talent
If you are truly going to be a data-driven business, data science should be a long-term commitment. I’m not saying you need to hire this potentially expensive person permanent full time, but if you’re considering a freelancer, you may want to look at some kind of retainer – particularly as high demand means there’s no guarantee the same talent will be available when you need their services once more.
Negotiating a retainer isn’t just about securing availability. You need the talent to get to know your systems, your business and your customers intimately, so when they’re working with the mountains of data, they will know instinctively which trends or connections are genuinely significant. It also means they’ll be able to get up to speed much faster and easier with each new project instead of starting from scratch with a new person every time.
Creating a data science culture
Beyond finding talent, building a data science capability also requires an internal culture that is ready to support it. There’s no point throwing money at someone, as well as the systems and the software they’ll need, if no one cares about the analysis they produce.
So, a data science capability needs to be championed from the top. Every time a decision is made, the CEO or executive mantra needs to be “Show me the data and the story that sits behind it.”
If you’re bringing in this talent on a project basis, you also need to create a freelance-friendly culture as well, to ensure the experience of working with you doesn’t dissuade the talent from accepting future projects.
Key Takeaways
- While data science and data analytics are related, the level of expertise and skill sets are different. Don’t assume your existing data analysis capability can begin delivering data science projects
- Demand for data science expertise is hot right now, so take time to plan your project to ensure it will be interesting enough to attract the best talent
- Data science requires more than just the expert talent. You also need to plan and ensure data hygiene throughout the business as well as get buy-in from the top down to instil a truly data-led culture
Get our insights into what’s happening in business and the world of work; interesting news, trends, and perspectives from our Expert community, and access to our data & trend analysis.
Be first in line to read The 360˚ View by subscribing below.
Hire exceptional talent in under 48 hours with Expert360 - Australia & New Zealand's #1 Skilled Talent Network.